Deepfake: Unearthing the Status Quo
Table of Contents
by:
Max Bineder and
Manuel Werka
Prologue #
It’s two o’clock in the morning and you wake up to your phone ringing. You, torn from sleep, reach out and pick up. Your boss is on the other end. He is currently somewhere across the globe and must have forgotten that time differences exist. His voice is filled with a subtle amount of panic and you, still dizzy, understand only half of his words. He demands that you get him some important client information before his next meeting, which is starting in two minutes. He says that he forgot his work laptop in his hotel room and you should send the information to his personal email instead.
- Would you, half asleep, send the files to him or would you question the legitimacy of the call?
Most of us would probably execute such requests, not thinking about the call being faked. The bad news is that the technology for such deception exists and such scenes will probably only get more common in the near future.
From Shallow to Deep Fakes #
What we are talking about here of course is deepfakes, which in the example above took the form of an audio deepfake. In this post, we will take a closer look at deepfake technology and its dangers and we will try to answer the following question:
- What is a deepfake and what makes it special?
Throughout history, a lot of images have been subjected to forgery. Oil paintings have been altered and faces have been swapped on analog photographs whilst being developed into prints. With the invention of Photoshop, the ability to fake photos became more accessible than ever before in history. Altering an image so that it was indistinguishable from reality, however, took a lot of effort and specialized training.
Exchanging faces in videos was even more difficult, as it was hardly possible to mimic the expressions of the face being replaced. Generally, it was a long process which could only be carried out by professionals. These fakes, so called shallow fakes, are not very convincing and in most cases real-time alteration was unthinkable.
Machine Learning to the Rescue #
Consequently, this problem can be solved by using machine learning to produce a deepfake. To utilize this technology, you will first have to create a face model, which is a dataset that contains the range of facial expressions the target’s face can make.
The quality of the fake, however, gets better the more the underlying model is trained. If a deepfake is not performed in real-time, the result can be further enhanced using audio and video editors. Depending on how professional you need your deepfake to be, creating one can take anywhere from a few days to several weeks.
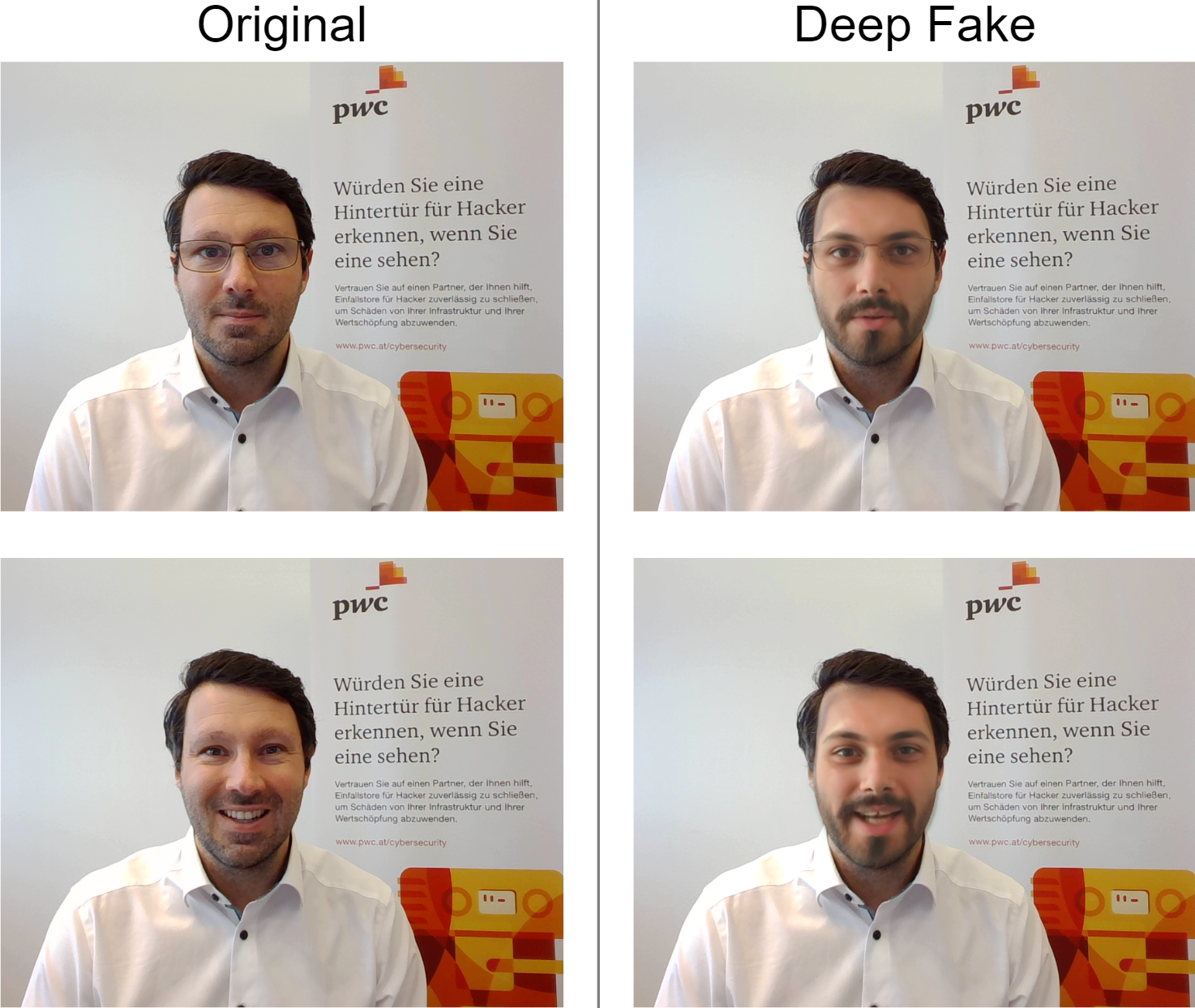
Deepfake Videos in Practice #
To generate a deepfake video, you must collect enough footage of the target person.
Ideally, this footage should show the target’s face from as many angles as possible and in a wide range of lighting. In addition, you must amass footage of as many of the target’s emotions and facial characteristics as possible. This will help replicate various facial expressions in the resulting deepfake video.
The resolution of the footage used to train the model should be as high as possible. The limiting factor of the face model resolution at present is the available hardware VRAM. For example, we managed to generate a resolution of up to 512x512 pixels for our face model using a NVIDIA GeForce RTX 3090 with 24GB of VRAM.
The deepfake generation process involves creating a face model of the target, which is based on the source footage. The destination video on the other hand has no special requirements other than it has to contain the face of the subject. To create a convincing result, the deepfake algorithm challenges itself by changing the head of the subject to that of the target.
As soon as the creator deems the results of the algorithm good enough, the face model is applied on every frame in the destination video that has the subject’s face in it. These images are then combined into the final deepfake video.
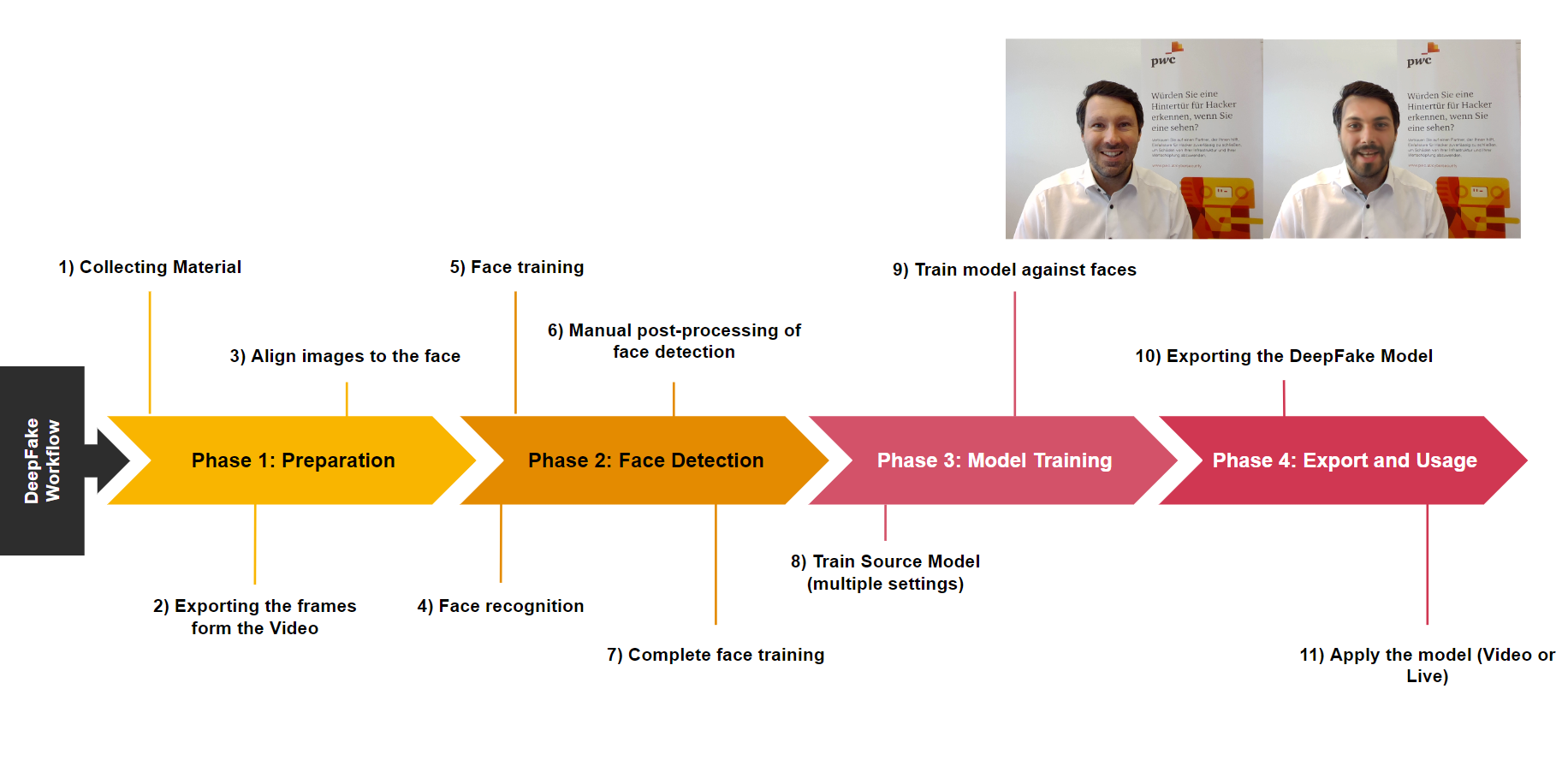
One limitation of most current deepfake technologies is that you can only change the subject’s face. You cannot change their hair, ears or body. The results will be the better, the more similar the target and the subject are. On TikTok for example, a character called deeptomcruise uses deepfake technology called metaphysic.ai to create very convincing results.
Live Deepfakes #
With the increase in hardware performance, it is now possible to apply a pre-created face model in real-time to a subject’s face. The face model creation process is quite similar to the video deep fake process even if the face model is not trained against a specific face in the destination video.
Live deepfakes are not as convincing as video deepfakes because you will not be able to fix the artifacts in the same way as you can via post editing or in this case, retraining and recreating.
Example on Youtube #
Depending on the hardware, the latency between the input and output video ranges from a few milliseconds to a second. The frame rate of the output video depends on the hardware, the latency, and the resolution of the input video. Using a high-end GPU it is possible to reach up to 25 frames per second with a 1920x1080 output resolution and an extremely low delay.
Audio Deepfakes #
Audio deepfakes do not have as high hardware requirements as video deepfakes. Therefore, many tools and services are available that can be used even by non-technical users.
To create an audio deepfake, you must first create a voice model. This model is trained with audio files of the target. These files should be as clear as possible and only contain the target’s voice. As with facial expressions above, the voice samples should cover as many linguistic variations and emotions as possible.
At present, there are two different types of voice deepfakes: text-to-speech and speech-to-speech. With text-to-speech audio deepfakes, you first specify a text which is then synthesized by the AI as an audio track with the target’s voice on it. With speech-to-speech deepfakes, a spoken voice is interpreted and recreated with the subject’s voice. Speech-to-speech deepfakes can also be created live.
Experienced audio deep fakers are able to create a nearly perfect deepfakes in approximately one to two weeks of any public person using only material available online (e.g. YouTube, social media).
Threats and Risks Related to Deepfakes #
Already for some time, almost anyone has been able to create relatively credible deepfakes. With more potential attackers, the number of deepfake attacks has risen sharply. Threat actors are willing to pay up to $16,000 for well made deepfakes on the Darkweb.
So far, the targets have mainly been political figures. In 2022, a deepfake video was distributed in which Volodymyr Zelenskyy, the president of Ukraine, called on his soldiers to lay down their arms.
Intellectual Property Theft #
In an act of intellectual property theft, an attacker could impersonate a customer or an employee to request sensitive company information from the employee. Both live audio and video deepfakes can be used for the attack. For a live video deep fake, the attacker would send a phishing email with a link to a video call. In this call, they would demand the employee for sensitive information about the company or the company’s customers.
CEO Fraud Utilizing Audio Deepfakes #
Financial assets can be stolen from a company using a similar approach. In this case, the attacker poses as a higher-ranking employee and requests an important bank transfer. The money is, however, transferred to the attacker’s account. Such an attack already took place in 2019, which resulted in approximately €220,000 being stolen from the victim organization.
Fake Video in the Name of a Person or a Company #
Deepfakes can also be used to damage the reputation of a person or a company. The attacker would for example simply create a deepfake video posing as a board member of a company. The video could show a board member engaging in illegal activities or sexual acts. Even if the video turns out to be a fake one, it could still damage the reputation of the company or the individual.
The risk of the first two cases (information and asset theft) can be reduced by training employees and performing further identity checks. Conversely, for every person who appears publicly, there is a risk that a deep fake video will be created of them. These videos must be discovered and marked as deep fake as quickly as possible. To ensure this, the process must be automated with the help of state-of-the-art deepfake detection technology.
State-of-the-Art in Deepfake Detection #
With the advent of fast deepfake generation, we will need fast and robust techniques to detect and flag them before users are duped on social media or in video calls.
But how do these techniques work and are they really as good as advertised?
When talking about Deepfake detection, most, if not all of them, can be categorized into one (or multiple) of the following methods.
Artifact Detection #
Artifact detection is the most basic form of a countermeasure, where an image is scanned for some rendering errors. Very obvious errors include things such as:
- missing glasses on one eye
- unrealistic looking reflections and lights
- or distorted faces.
Such obvious examples are often easily caught by eye and aren’t really present in modern deepfakes. More subtle rendering artifacts are uneven spots on the image, which look like small raindrops.
Artifact detection works very well with earlier deepfakes, but does not catch most of the state-of-the-art ones. This method is good at flagging retouched images, but retouching is so commonplace for most portraits that this method alone will not suffice. That is why it is often used in combination with other detection methods due to its rather low computational performance requirements.
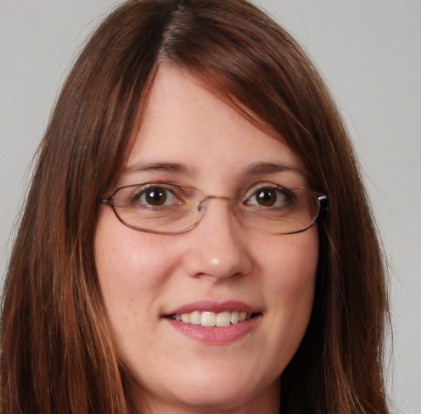
Multi-Regional Detection #
The multi-regional detection method is usually used for detection of disparate objects within a frame. A slightly modified version of this method can be used to spot deepfakes by analyzing the proportions of faked faces. In extreme cases these inconsistencies can be observed by eye and they look like a poorly edited photo, where a portion of a face was just pasted onto a head.
With this technique it is also possible to spot slight errors in the target’s viewing direction. What we mean by this is that the direction of the head does not correspond with the direction of the inner face. Such errors often originate from poor source data curation.

Analysis of Biological Signals #
As the name suggests, this detection method relies on the analysis of a target’s biological signals.
These signals include:
- a heart-rate measurement using the difference in skin coloration (photoplethysmogram)
- an analysis of various muscle movements of the face.
This detection method is rather elaborate and requires a clear (meaning non-blurry), high resolution image to get reliable results.
Analysis of Audio and Video Synchronicity #
The analysis of audio and video synchronicity is not as straightforward as one might think, since it does not simply check if the audio is in sync with the video. How it actually works is that the tempo and pitch of the voice on the audio track is compared against the movement of the target’s facial muscles on the video track. A natural flow of speech presents muscle movement, which conveys vocalizations and emotions present in the audio.
This detection method is actually the only one which uses both audio and video to determine the authenticity of a given clip.
Detection Challenges #
As with deepfake generation, its detection relies on the quality and quantity of the training sets. Conversely, the best methods trained with poor data will not perform worse than poor methods trained with high quality data. Most of the methods are open source, especially the ones suggested in scientific papers.
There is nothing wrong with that in itself, but the problem seems to be that each author is trying to convince you that their solution is the very best. Furthermore, comparison between methods is made difficult, since either the training set is not clearly defined or the detection method itself is not clearly aligned with similar detection methods.
In an ideal world you could use the same training set and test data for all methods and pick the best one. Furthermore, it does not help that most of these methods use older versions of programming languages and frameworks which are not supported or even downloadable anymore. The comparison between commercial solutions is even harder, since these are mostly complete black boxes to the end-user and are only described by the usual buzzwords such as AI or Deep Learning.
Validation of Authenticity #
As with any new technology which is used by malicious parties, it will probably end up in a game of cat and mouse where threat actors are trying to stay one step ahead of the defenders and vice versa.
One proposed solution to this problem is the introduction of an external authenticity check, which basically boils down to using versioned video and audio files in a similar fashion as published source code.
If widely adopted, it would be possible to look up the full history of a file and find out who modified it. This certainly would not stop deepfakes on its own, but if done right it could offer individuals a way to add a modicum of trust into the authenticity of their content.
Deepfake Trends #
The term deepfake is probably already known by a lot of people due to some kind of media coverage. Due to sinking costs of the required hardware and continuous advances in the deepfake generation software, the topic will become even more commonplace.
It is likely that many more parties with malicious intent will use deepfakes to extort their victims. Therefore, it will be necessary for governments to implement legislation on how to deal with realistic fakes. These laws should include key points such as how a person can be held accountable for falling for a deepfake.
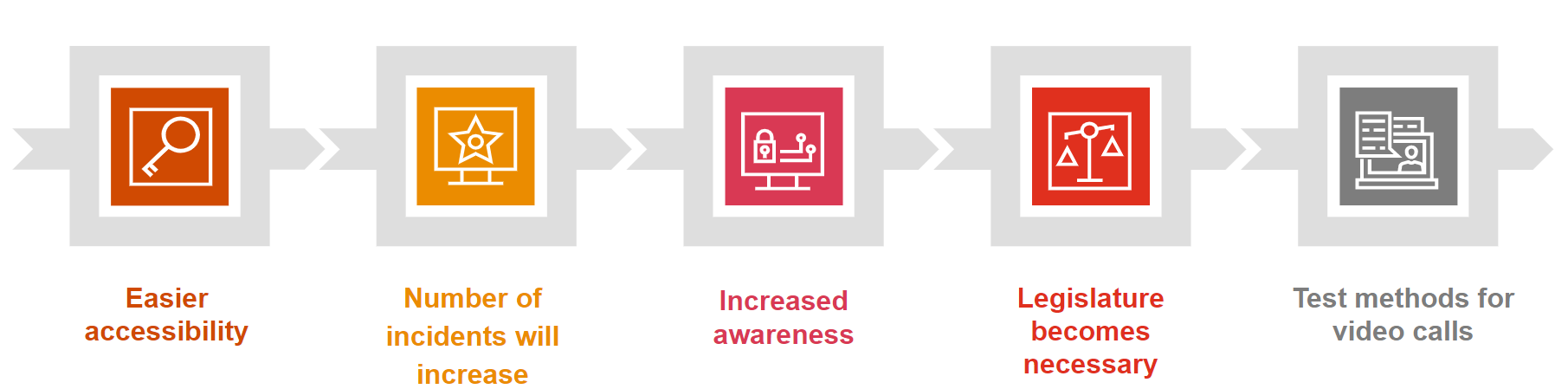
Companies should also implement guidelines on how to deal with deepfakes and increase awareness on this topic. This is especially true for media outlets which have to determine if events posted on the Internet are trustworthy, particularly concerning sensitive topics. Presumably somewhere in the near future, the companies behind the most popular video conferencing software will implement some kind of deepfake detection mechanisms in their products.
In addition, third parties will offer easy to use deepfake detections tools, possibly included in EDR suites, as is already common practice in phishing detection. In the long term, the deepfake hype will flatten out due to increased awareness by most people. A similar effect can already be seen concerning simple video editing, where some implausible event is instinctively seen as doctored by the general public.