Audio Deepfakes and Voice Cloning - When you can't trust your own ears
Table of Contents
by:
Manuel Werka
In a world where familiarity brings comfort and security, the rise of Deepfake technology poses a concerning threat. The voices of loved ones, colleagues, and trusted figures have long served as pillars of reassurance in our lives. However, the emergence of audio deepfakes, also known as voice cloning, challenges this sense of safety by enabling the replication of any person’s voice with alarming accuracy. This technological advancement presents significant risks to individuals, businesses, and society as a whole.
What are Deepfakes? #
The term deepfake refers to the realistic manipulation of visual and audio content. Deepfakes have attracted significant attention due to their potential to deceive, manipulate, and undermine the authenticity of digital media. The rise in hardware performance makes these techniques accessible to the broad public.
The generation of fake media that average computer users cannot identify with video and audio gathered from video platforms or social media is possible for anyone with a mid to high-end computer. This enables attackers to weaponize this technology for their malicious purposes. Although there are many types of deepfakes, this write-up focuses on audio deepfakes.
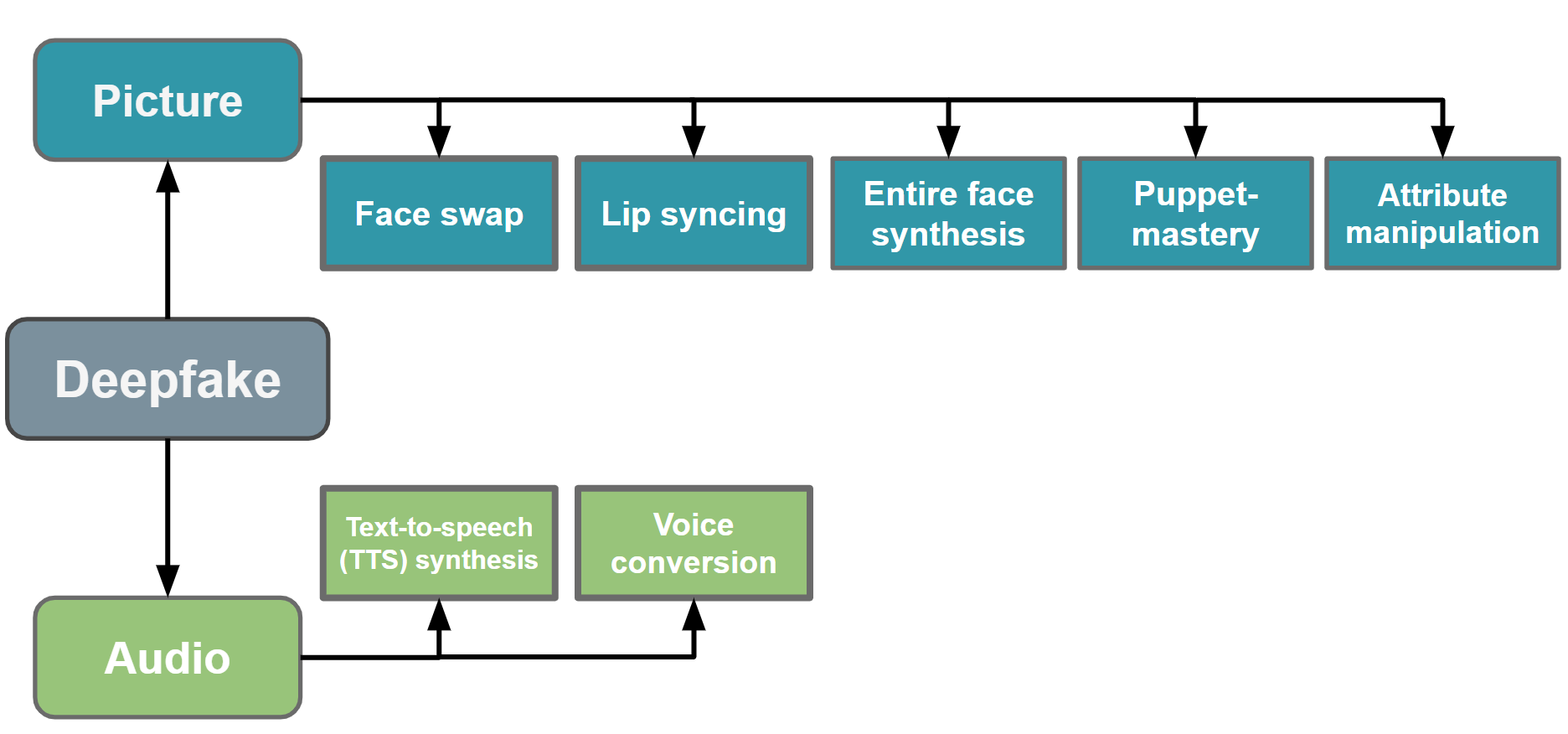
Understanding Audio Deepfakes #
There are two types of audio deepfakes:
- Text-To-Speech (TTS)
- Voice Conversion.
These techniques differ in their use and applications.
Voice Conversion #
Voice conversion technology alters an input voice to sound like the target voice. The result is audio with the characteristics, e.g. pauses or pronunciation of the source speaker that sounds like the target speaker.
General Model Creation #
For both types it is necessary to first create a model. A model is created by using mathematical equations on a large set of data to find specific patterns. These patterns can then be applied to new data using said model. In the case of audio deepfakes a model is trained by using large amounts of audio clips of people talking. During the learning process, the model learns from this data to replicate how humans sound.
The difference between the TTS and voice conversion is that TTS requires the words associated with the voice, whereas voice conversion just replicates the sound of the speaker. This means that a TTS model has to be trained for each language individually, whereas for voice conversion only one model is required, which can be used for all languages.
Live Use of Audio Deepfakes #
When it comes to the live use of audio deepfakes, at present only voice conversion is feasible. The combined time of typing the text and generating is too long for a natural conversation. Voice conversion allows for a live deepfake with around 300ms delay. This delay is necessary to adjust the pitch of the audio, so it sounds nearly the same for the whole conversation.
Audio Deepfake Creation #
Creating a specific audio deepfake model is not as hardware dependent as a video deepfake model. It is possible to create a good audio model of a person in around 1.5 hours using a RTX4090 GPU. Before a specific model can be trained, it is necessary to create a data set consisting of audio material from the target person.
Dataset Creation #
The most important part of the deepfake creation is data collection. The deepfake can only reproduce what was available in the dataset. Therefore, it is important that the audio clips in the dataset are as clean as possible. This means that there shouldn’t be any disruptive sounds in the material as these will be in the final model and thus in all audio deepfakes that are created with this model.
The dataset’s size should range from a few seconds to over 10 minutes. While larger datasets generally yield better results, adding low-quality material is discouraged as it may degrade the model’s performance.
Creating the Actual Deepfake #
The created dataset is used to generate a specific deepfake model for the person that is to be faked. For most frameworks, the audio is provided in a folder for the framework to use. After the training process, the frameworks require an input to combine with the general model for human audio creation and the specific model for the voice of the person that is to be faked.
The input for the deepfake creation differs between the two types of audio deepfakes. TTS requires text input to create the audio, where voice conversion requires speech. The creation time differs by the quality and framework used. The following table describes the time necessary to create a one sentence audio sample with the TTS framework Tortoise TTS on different GPUs with different quality:
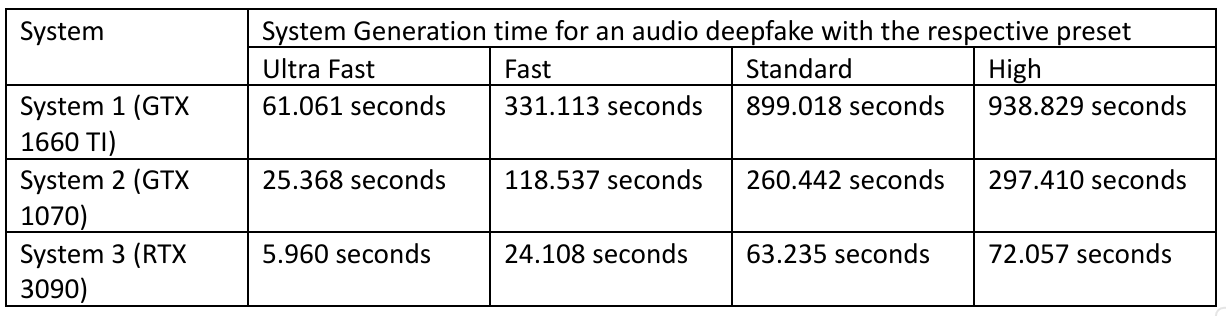
It is possible to generate live audio deepfakes with the help of a Voice conversion framework such as RVC and at least an RTX3090.
Threats, Risks and Detection #
Especially the use of live audio deepfakes poses a threat to society, individuals and companies alike.
Fraud, Scam and Industrial Espionage #
Deepfakes can be used to deceive individuals or organizations, leading to financial losses and fraudulent activities. Attackers may create fake audio calls to trick people into providing sensitive information or making unauthorized transactions. This happened in a recent case in Hong Kong where 25 Million USD were paid to fraudsters during a deepfake call.
Disinformation and Public Distrust #
Deepfakes spread false information, damage business reputation, losing customers, and eroding trust. Deepfakes manipulate elections by spreading fake audio or video recordings of candidates, influencing voter opinions, and undermining the democratic process.
This impacts businesses as political stability and policies directly affect the economic environment. An example is the recent deepfake of the German chancellor where he said that he will ban another political party.
Identity Theft #
Deepfakes can be used to impersonate individuals, leading to fraudulent activities and unauthorized access to sensitive information. This can result in financial losses, reputational damage, and legal consequences for businesses or individuals.
Extortion #
Deepfakes can be used as a tool for extortion, where attackers threaten to release manipulated audio or video recordings unless a ransom is paid. This can cause financial damage to private persons.
Can an audio deepfake be detected? #
Detecting good audio deepfake attacks without a tool is also very hard. Especially when the attacker also uses caller id spoofing. This is a technique that allows the attack to mimic the phone number of the deepfaked person. The phone of the victim matches the phone number to the contact and displays the correct name. In addition with the voice of the deepfaked person it is nearly impossible to detect this attack.
So what can you do? #
An attacker always has a goal during the call. Often they are trying to pressure their victim to act fast on their objective. It is important to stay calm and think about the following things:
- Is this the normal behavior of the caller?
- Does the caller’s request make sense?
If you are in doubt, try to confirm the caller’s identity by a second factor for example by calling them back or writing a text message or even schedule an actual meeting in the real world.
Deepfake Trends #
The concept of deepfake has likely entered the mainstream consciousness through media exposure. With the decreasing costs of necessary hardware and the continual advancement of generation software, its prevalence is set to increase further. Unfortunately, this opens the door for malicious actors to exploit deepfakes for extortion and other nefarious purposes.
Consequently, it becomes imperative for governments to enact legislation addressing the handling of realistic fakes. Such laws should delineate accountability for individuals who fall victim to deepfakes. Moreover, companies must establish protocols for dealing with deepfakes and raise awareness about the issue, particularly pertinent for media outlets vetting online content, especially on sensitive subjects.
In the foreseeable future, major video calling software providers may integrate deepfake detection mechanisms into their platforms. Additionally, third-party tools for easy deepfake detection may become commonplace, potentially integrated into antivirus suites, akin to phishing detection tools.
Credits #
- Hero image by Ben Koorengevel on Unsplash.